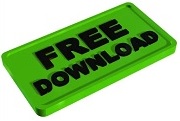

For most problems of interest, this dynamic programming solution, albeit exponential, for reasons we've discussed in the past, will be a significant improvement over naive brute force search. The dynamic programming algorithm takes time proportional to n, the number of items, times capital W, the knapsack capacity. It's going to take time proportional to 2 to the n, where n is the number of items. Now, you've brute force search, enumerating over all possible subsets of items. Our dynamic programming solution to the knapsack problem can be viewed as a contribution in this direction.

But still to have running time qualitatively better than what you'd achieve with naive brute force search. The third strategy is to insist on a correct algorithm, and being an NP-complete problem, you're then expecting to see exponential running time. I'll have more to say about those in a second. The second approach is the design and analysis of heuristics, algorithms which are not guaranteed to be 100% optimal. For example, if it's comparable to the number of items. Namely, knapsack instances where the knapsack capacity is small. A dynamic programming solution for the knapsack problem identifies one such special case. Even otherwise, it's useful to have these subroutines lying around, perhaps with a little bit of extra work, you can reduce your problem instances to one of these computationally tractable special cases. If you're lucky, the instances relevant for your application will fall squarely into one of these computationally tractable special cases. The first strategy is to identify computationally tractable special cases of the NP-complete problem that you're grappling with. All of these are relevant for the knapsack problem. Let's briefly review the three strategies for dealing with NP-complete problems. As a case, study we're going to revisit the knapsack problem that we thought about earlier in the dynamic programming section. This sequence of videos is about the design and analysis of heuristics, algorithms which are generally guaranteed to be fast but not guaranteed to be 100% correct.
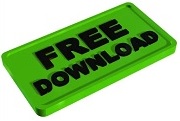